Learn about the benefits of constructing a multi-year internship from a MLIS student's perspective.
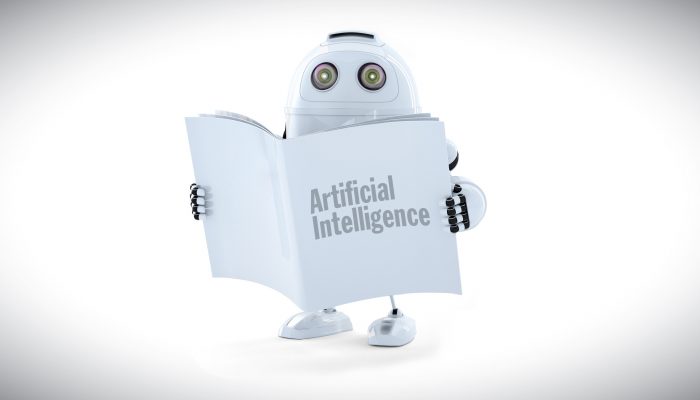
Explainable Artificial Intelligence (AI)
by Mike Ridley
If AI are so smart, why can’t they explain themselves? Autonomous, intelligent systems are becoming ubiquitous. If you use Google, Facebook, Netflix, or a host of other services, you’ve been using artificial intelligence (AI).
In many areas AI have achieved human level performance; in some areas, they have gone beyond that. It’s not just gaming (AI regularly beat master players of Chess and Go), healthcare AI are superior at radiology, aspects of oncology, drug development, and many other areas.
All good, right?
Well, sort of.
It turns out that advanced AI techniques, such as deep learning (a type of neural network), are extraordinarily powerful at making predictions and recommendations with very complex data, they just can’t explain to us how and why they came up with the decision.
They are inscrutable. Black boxes.
Geoffrey Hinton (University of Toronto), often called the godfather of deep learning, explains: “A deep-learning system doesn’t have any explanatory power. The more powerful the deep-learning system becomes, the more opaque it can become.”
Are you going to trust the recommendation of a medical AI if it can’t explain to you, or your doctor, why it determined the diagnosis and the treatment regime? No, I didn’t think so.
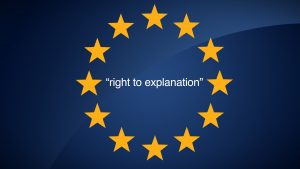
The European Union agrees with you. This year they enacted the General Data Protection Regulation (GDPR). The GDPR is primarily about personal data protection but it includes what is being called a “right to explanation” for any decision about you made by an “algorithmic agent” (an AI system).
Again, all good?
Well, sort of.
If you live in the EU, AI systems are now required to provide an explanation. It’s your right after all. However, AI still can’t do it. They are still opaque, black boxes.
Needless to say, we have a problem.
Which brings me to some of the research I’m doing as part of my PhD program at the Faculty of Information and Media Studies (FIMS), Western University. I’ve been looking into the various ways AI might be able to explain themselves, and there are some promising options.
But first, why does this matter to libraries?
Andrew Ng, a leading researcher in the field, calls AI “the new electricity.” Think less about know-it-all robots in the workplace, and more about smart toasters and intelligent Word documents. AI will be invisible but omnipresent. The Internet of Things (IoT) is going to be very clever. AI will be embedded in the tools we use as well as in our collections. And perhaps even something more.
Chris Bourg, Director of Libraries at MIT, posted “What happens to libraries and librarians when machines can read all the books?” to her Feral Librarian blog on March 16, 2017. Provocatively, she recommended that “we would be wise to start thinking now about machines and algorithms as a new kind of patron.”
Let that sink in: a new kind of patron.
Treating AI as a patron means thinking about how we can serve them and help them improve. Part of that is helping them explain their predictions and recommendations.
The options for “explainable AI” (often referred to as XAI) group into three general areas: proofs, validations, and authorizations. Proofs are explanations that are testable, demonstrable, traceable, and unambiguous. Validations are explanations that confirm the veracity of the AI based on evidence or argumentation. Authorizations are explanations as processes, typically involving third-parties that provide an assessment or ratification of the AI. All three of these options might relate to the algorithmic model, its operation in specific instances, or the process by which it was created.
Proofs are powerful but rare, and applicable to only certain types of AI. Let’s leave them for now and look at examples of a validation and an authorization.
“Feature audit” is a form a validation that examines the characteristics (“features”) that an algorithm is interpreting. By isolating specific features, it is possible to determine their impact on the outcome thereby explaining the prediction. Feature audit is a good way to expose bias and discrimination in the data and the algorithmic model.
The most common strategy for explainable AI is some form of authorization. Most authorization strategies call for the establishment of a regulatory agency with legislated or delegated powers to investigate, certify, license, and arbitrate on matters relating to AI and algorithms including their design, use, and effects. One US researcher, Andrew Tutt, considered the Food and Drug Administration as a model and recommended an “FDA for algorithms.” No such proposal has been contemplated in Canada.
Canada is a leader in AI research and deployment. Federal and provincial governments are pouring money into AI think tanks, accelerators, and startups. There is a lot of money, jobs, and economic heft at stake. There is also intense international competition. For too long AI has been the sole domain of technologists. As the power and implications of AI become clear, it is past time for other disciplines to become involved.
Libraries have typically “humanized” technology by making it accessible and user-centric. If Chris Bourg is right, and I have every reason to believe she is, AI is a major opportunity and challenge for the library community. Explainable AI is just one area where we can make a difference.
Michael Ridley a Librarian at the University of Guelph and a PhD student at the Faculty of Information and Media Studies (FIMS), Western University. Ridley is a former Editor-in-Chief of Open Shelf (2014-2017). He can be contacted at mridley [at] uoguelph.ca and on Twitter @mridley.