The E-Learning in Libraries Symposium, organized by library professionals, shares advancements in e-learning initiatives across various libraries in Canada. In its 10th year, it continues to promote collaboration and community among professionals adapting to digital learning landscapes, hybrid work environments, and AI integration.
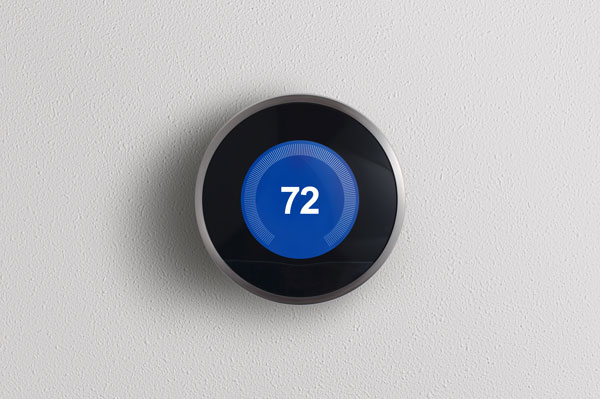
Different Views of “aboutness”: Rise of the Machines?
We all see things a little differently. Unique perspectives can help to feed curiosity and innovation. But in an era of information overload, differing viewpoints may cause frustration for information producers and consumers alike. Authors and publishers want their works to get noticed, while readers must navigate the plethora of publications and focus on those that are the most pertinent to their needs. Given the various possible interpretations of a single publication, who can provide the most useful description of its contents?
Traditionally, readers have relied on professionally-produced indexes or author-recommended keywords, and these methods have been studied in some depth. But Web 2.0 ushered in new possibilities, which led to investigations into the effectiveness of social tagging by readers, and comparisons of this method to conventional approaches. As technologies continue to evolve, we suggest that it is also worth exploring whether automatic tools can add value to the indexing process. In particular, we investigate whether TermoStat—an automatic term extraction tool initially conceived for use by terminologists and translators—can be usefully adopted for indexing.
Turn up the TermoStat!
Many term extractors rely on frequency counts to determine whether a particular term seems relevant. Although frequency can certainly be a potential indicator of a word’s importance in a text, it is not sufficient as a sole measure of “aboutness”. In addition to a frequency count, TermoStat calculates a specificity score by comparing the contents of a specialized text against a much larger general reference corpus to identify those words in the specialized text that are unusually frequent as compared to their frequency in a larger general reference corpus. In other words, TermoStat’s specificity score is a measure of the frequency of disproportionate occurrence. Curious to know how the results of this type of automated objective textual analysis would compare to the more subjective content description techniques that draw on intuition, we devised a small experiment.
The corpus used for this pilot study consisted of four chapters taken from a scholarly edited collection in the discipline of Translation Studies. The volume has a back-of-the-book index that was produced by a professional indexer (P). The author of each individual chapter also provided a list of keywords corresponding to that chapter (A). Next, we asked a PhD student in the discipline to read each chapter (without having access to either the back-of-the-book index or to the author recommended keywords) and to supply a list of keywords for each chapter, thus providing the reader’s perspective (R). Finally, the TermoStat tool was used to generate a keyword list for each chapter automatically (T).
While previous researchers offered their own analyses about the effectiveness of different types of descriptor lists, we approached this question from a different perspective. We asked students and professors—people who might actually be seeking these texts—to weigh in on the matter. Who will come out on top in the battle to inform potential readers about a document’s content? Will it be an information professional, an author, a peer… or an automated tool?
Battle of the Descriptors
To explore whether the target audience found certain types of descriptor lists to be more useful than others, we conducted an experiment where we asked 24 graduate students and professors who conduct research in the field of Translation Studies to read one of the chapters without reference to its related descriptor lists. After reading the chapter, they were shown (in random order) the four corresponding lists as produced by:
(P) – professional indexer
(A) – author
(R) – reader
(T) – TermoStat automatic term extractor
After consulting the four lists, participants were asked to rank them from best to worst according to how well they perceived that each list described the contents of the chapter. The survey tool calculated a weighted average for each list. The chart shows the amalgamated results for the four chapters. Overall, the participants preferred the list produced by the reader (R), followed by the list produced by TermoStat (T), by the authors (A), and finally, by the professional indexer (P).
All Together Now!
Owing to the small number of participants and texts, the data for the pilot study cannot be considered conclusive. Nonetheless, some possible trends were observed:
- The descriptor lists produced by the professional indexer were ranked last on average.
- The descriptor lists produced by TermoStat were never ranked last, and in the amalgamated results, they placed second overall.
- The descriptor lists produced by “non-experts” (i.e., readers and TermoStat) were never ranked last, and so appears it appears that the non-experts in this pilot study produced lists that were perceived as being more helpful than the lists produced by “experts” (i.e., the professional indexer and the authors).
Further work is needed to generate a larger set of data to confirm, refute, or nuance the above observations. Moreover, our intention is not disparage the work of experts, who certainly bring added value to the task of making texts findable. However, the fact that the lists produced by TermoStat were consistently ranked quite highly would suggest that automated tools have something to contribute, and their lists of candidate descriptors could perhaps be a useful starting point for an indexer. As a final observation, we note that the various lists produced for this pilot study appear to be complementary (i.e., there was not a lot of overlap in content). Therefore, a collaborative approach to identifying content descriptors could be the most beneficial approach of all.
Lynne Bowker is a professor at the University of Ottawa’s School of Information Studies. Email: lbowker [at] uottawa.ca.
Rebecca Mackay is a student in the BA in Translation program at the University of Ottawa.
Deni Kasama holds a PhD in Linguistics from UNESP (Brazil), and he currently teaches linguistics and translation at UNILAGO, Brazil.
Jairo Buitrago Ciro is a recent graduate of the University of Ottawa’s Master in Information Studies program.